CW3E Student Helps Facilitate Virtual Conference on Sustainability and the Global Pandemic
April 27, 2020
On Earth Day, nearly 100 researchers, students, and educators joined across time zones, career stages, and academic backgrounds for the inaugural E-Conference on Conservation, Sustainability, and the Global Pandemic.
This 100% virtual conference was organized to allow students, postdoctoral researchers, and early career professionals the chance to share their research on a global platform, and to offer insights and hopeful words from researchers and educators further in their careers. Participants joined the meeting on Blackboard Collaborate from Australia, Peru, the UK, the U.S., and other locations. They posed questions in the chat box and on Twitter using #CSGP2020.
The half-day event was organized and facilitated by Dr. Gillian Bowser and Sarah Whipple (Colorado State University), Dr. Brian Forist (Indiana University), Dr. Andrew Ramsey (University of Derby, UK), Dr. Susie Ho (Monash University, Australia), Dr. Javier Ñaupari (La Molina University, Peru), Dr. Sarah Green (Michigan Technological University), Dr. Pamela Templer (Boston University), CW3E student Tashiana Osborne, and others.
Following the opening keynote address by Dr. Richard Primack of Boston University, Osborne moderated an engaging 12-member panel focused on pandemic impacts on natural science fields, education, climate, policy, conservation and biodiversity efforts, global Sustainable Development Goals and negotiations (United Nations COP26).
Bowser, Green, Whipple, Ho, Ramsey, Primack, Templer, Ñaupari, Forist, and Osborne sharing insights and calls to action, as questions and comments stream in through the chat box and Twitter (Screenshots from Blackboard Collaborate)
After the plenary panel, participants were invited to virtual coffee breaks on various Slack channels before returning for poster sessions. Poster presentation topics ranged from COVID-19 impacts involving decreases in new greenhouse gas emissions since the start of the pandemic and increases in residential and hospital waste, to warming global temperatures contributing to decreases in Atlantic Cod populations along Norway’s coast, changes in land cover and use in micro-basins of Peru, and more. Osborne worked alongside Dr. Green to lead a session on atmospheric science and biogeochemistry topics.
Link to student presentations: https://derbyuni.padlet.org/a_ramsey2/padletofpadlets
Poster and pre-recorded video presentations on various natural science, sustainability, and conservation-related subjects involving mountain ecosystems, atmospheric matters, and more (Screenshot from Padlet)
In the end, this completely virtual, multidisciplinary, international conference proved to be both informative and uplifting. It served as a learning experience for all involved, especially during a time where physical distancing has been paramount and the future seems particularly uncertain.
CW3E Welcomes Peter Yao
April 27, 2020

Peter Yao joined CW3E as a Research Data Analyst in January 2020. Before joining Scripps he worked in several different fields, including robotics, software development, and automotives. He earned a B.S. in Biomedical Engineering from Johns Hopkins University in 2015, and an M.S. in Mechanical Engineering from the University of Michigan in 2017. At U-M, his Masters research involved developing a numerical model of the pressure distribution within an engine cylinder during stochastic pre-ignition. After graduating he worked as an engineer at General Motors, where he designed clutch control algorithms for automatic transmissions.
Peter made the leap to climate science when he joined the Great Lakes Integrated Sciences & Assessments Center (GLISA) as a Research Associate in 2019. He supported the group’s efforts to build climate resilience in the Great Lakes region by helping develop many of the observational and modeling datasets used in their work. At CW3E he will help the center maintain robust observational datasets and support numerical weather prediction model validation efforts. He is very excited for the opportunity to continue growing both his computing skills and climate science knowledge.
CW3E Publication Notice
PERSIANN Dynamic Infrared–Rain Rate Model (PDIR) for High-Resolution, Real-Time Satellite Precipitation Estimation
April 23, 2020
A team from the Center for Hydrometeorology and Remote Sensing (CHRS) at UC Irvine led by Dr. Phu Nguyen, along with CW3E director Dr. Marty Ralph, recently published a paper entitled “PERSIANN Dynamic Infrared–Rain Rate Model (PDIR) for High-Resolution, Real-Time Satellite Precipitation Estimation” in the Bulletin of the American Meteorological Society (BAMS) where they introduce a new real-time satellite quantitative precipitation estimation technique – PDIR model. PDIR was created as part of the Atmospheric River (AR) program, a multi-organizational, multi-discipline program focused on becoming more resilient to extreme precipitation. A key strategy of the AR Program is to identify and fill observational data gaps to better prepare for extreme precipitation events, particularly ARs. As part of the AR Program’s observational strategy, the PDIR technique improves upon the current state of real-time satellite retrievals of precipitation totals over the US West Coast, which are imperative tools for natural hazard agencies combating AR-related flooding.
PDIR is based on the framework of the PERSIANN-Cloud Classification System (CCS) algorithm, which is in turn based on the original PERSIANN framework. PERSIANN, PERSIANN-CCS, and PDIR are satellite-based data-driven precipitation estimating systems that work primarily by establishing a link between geostationary-derived cloud-top temperature from infrared and rain rate using machine learning techniques. PDIR was created for the purpose of developing a high-spatiotemporal-resolution, near-real-time precipitation dataset that solely utilizes infrared data as input. PDIR advances the framework of the PERSIANN-CCS system by improving the capture of warm precipitation by adapting higher temperature thresholds, expanding the cloud classification system to include monthly sets of cloud types, and utilizing gridded monthly rainfall climatology data to create a dynamically shifting cloud top temperature–rain rate curve relationship.
PDIR was shown to accurately mimic the observed spatial patterns of rainfall over the western Contiguous U.S. (CONUS), notably the high rainfall amounts over the Cascade Range and Sierra Nevada. PDIR’s noteworthy performance in capturing the western CONUS’s rainfall, especially with high intensities and over mountainous regions, suggests a level of success in adapting to the challenges of the differing rainfall regimes that are intrinsic to the area, i.e. brightband, nonbrightband, and hybrid (seeder-feeder) rainfall. Of particular interest is that PDIR yields rainfall estimates for extreme rainfall events like ARs that are comparable to the nearly instantaneous, multi-sensor product, Stage II. Furthermore, the spatial patterns of PDIR’s precipitation estimates in mountainous regions better mimic those recorded by Stage IV than those shown by Stage II. The algorithm’s success at these scales suggests that PDIR contains the spatiotemporal richness and near-instantaneous availability necessary for rapid hazard response while showing potential to be useful for hydrologic and water resources applications, of which the latter has been a major weakness of infrared-based algorithms to date. As the implications of this study are valuable to a global audience, PDIR’s future direction is chiefly focused on its global implementation.
Figure 1. The dynamic cloud-top brightness temperature (Tb)-rain rate (RR) model: In PERSIANN-CCS, clouds that are identical in shape, size, temperature distribution, and all other defining characteristics but spatial location have completely identical RR readings. However, in PDIR, the underlying climatology causes shifts in the Tb-RR curves that characterize cloud-based models like PERSIANN-CCS and PDIR. In PDIR, drier climatologies such as the Mojave Desert (MD) cause a leftward shift in the Tb-RR relationships, which results in lower precipitation rates than at identical temperatures in moderately wet regions such as California’s Central Coast (CC), while wetter climatologies such as the Klamath National Forest (KNF) cause a rightward shift, which causes an increase in estimated precipitation rates for identical temperatures.
Nguyen, P., E. J. Shearer, M. Ombadi, V. A. Gorooh, K. Hsu, S. Sorooshian, W. S. Logan, and M. Ralph, 2020: PERSIANN Dynamic Infrared–Rain Rate Model (PDIR) for High-Resolution, Real-Time Satellite Precipitation Estimation. Bull. Amer. Meteor. Soc., 101, E286-E302, https://doi.org/10.1175/BAMS-D-19-0118.1
CW3E Event Summary: 5-10 April 2020
April 15, 2020
Click here for a pdf of this information.
Unsettled weather pattern brings record precipitation to Southern California
- Multiple episodes of heavy rainfall during 5–10 Apr were associated with a cutoff low near the U.S. West Coast
- More than 2 inches of precipitation fell over a large portion of Southern California, with the highest amounts (> 6 inches) in the Transverse Ranges and northern San Diego County
- Significant snowfall (> 12 inches) occurred in the higher elevations of the Transverse Ranges
- Intense rainfall resulted in flash flooding throughout San Diego County on 10 Apr
Click 500-hPa Absolute Vorticity or IWV image to see loop of GFS analyses
Valid 0000 UTC 5 April – 1200 UTC 11 April 2020
|
|
|






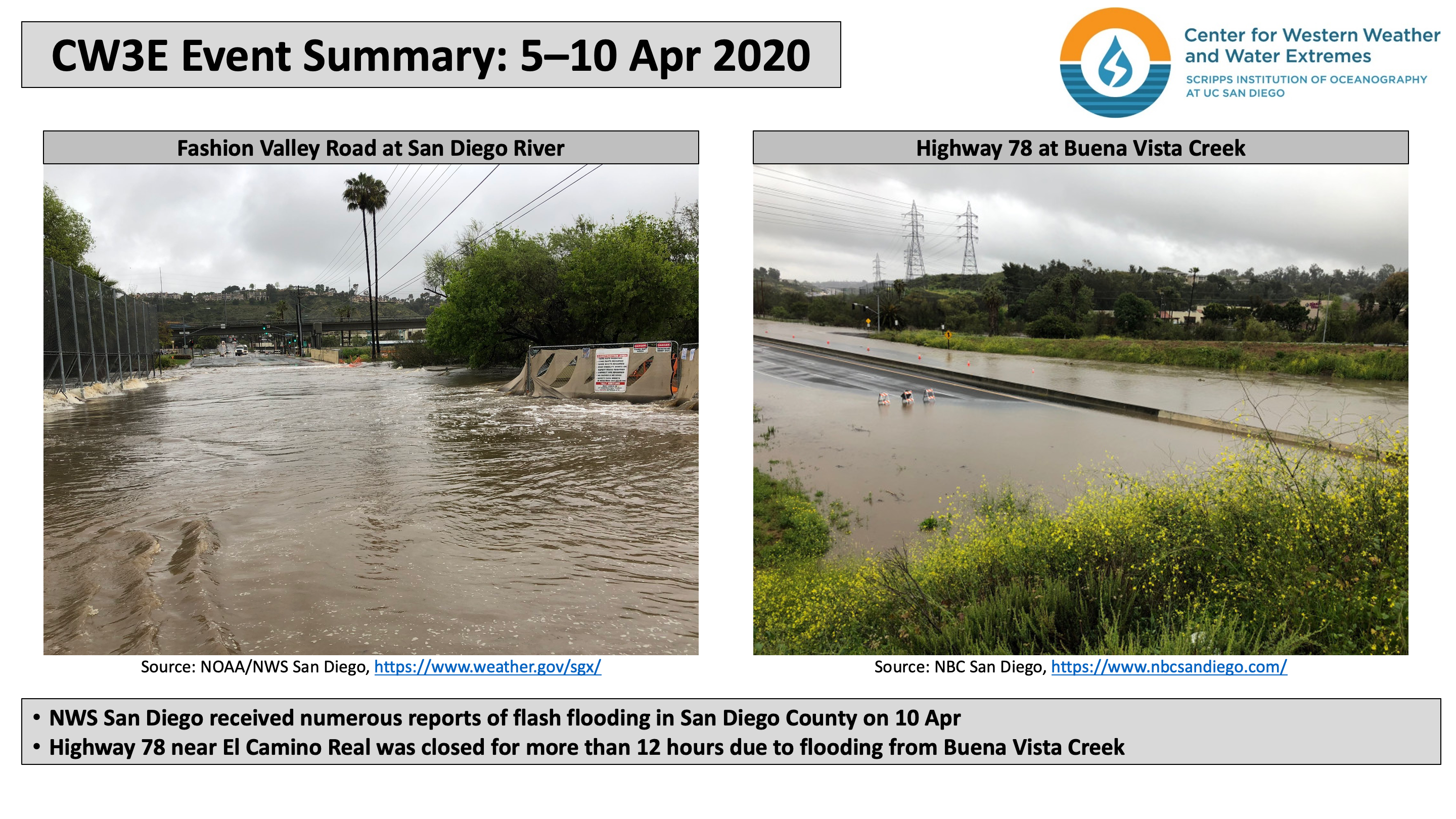
Summary provided by C. Castellano, C. Hecht, J. Kalansky, B. Kawzenuk, and F. M. Ralph; 15 April 2020
CW3E Publication Notice
Quantifying the Spatial Variability of a Snowstorm Using Differential Airborne Lidar
April 13, 2020
CW3E post-doc, Ty Brandt, lead a recently published paper presenting a new methodology to measure snowfall and compared to other SWE estimates. Accurate measures of snowfall are important because California depends on snow accumulation in the Sierra Nevada for its water supply, and accurate estimates of the total water stored in the snowpack are essential to water resource management. Presently, snowfall is measured by a combination of snow pillows, snow courses, and rain gauges. However, the scarcity of these measurements, particularly at high elevations, and their associated errors impose a limit on hydrologic forecasting. To reduce forecast errors, we require high-resolution, spatially-complete measurements of precipitation amount and phase. In snow-dominated watersheds, remotely sensed snow depth and snow water equivalent (SWE), with retrieval time scales that resolve individual storms, may ameliorate the existing data gap. Co-authors on the paper included CW3E’s Forest Cannon and Daniel Steinhoff, as well as collaborators from U.C. Santa Barbara, NASA Jet Propulsion Laboratory, the National Snow and Ice Data Center, and UCLA.
Since 2013, NASA’s Airborne Snow Observatory (ASO) has used airborne lidar to accurately measure snow depth in California’s Sierra Nevada for the purposes of advancing streamflow forecasting. However, in early April 2015, two flights 6 days apart happened to bracket a single snow storm. The research utilized these flights to develop a methodology using airborne lidar to directly measure the spatial variability of accumulated snow as a proxy for “snowfall” at a 50 m spatial resolution. In an end-to-end analysis, the researchers also compared gauge-interpolated and dynamically downscaled estimates of precipitation for the given storm with that of the ASO accumulated SWE (see figure below). This particular event proved an interesting test case due to the storm’s moderate snowfall generated by high amounts of dynamical forcing rather than strong orographic enhancement. Results highlighted that statistically-interpolated precipitation estimates were overly-reliant on climatological patterns of orographic enhancement, and that although ensemble WRF simulations accurately represented the event’s evolution and precipitation mechanisms, their snowfall distributions were highly sensitive to model parameterization choices.
While the April 2015 storm was a peculiar end-member for storms in the Sierra Nevada (quite distinct from the more impactful atmospheric rivers and their predominant orographic influences) it did serve as an important example to demonstrate the potential of differential lidar observations to quantify snowfall. By directly observing the snowfall distribution, ASO data provided validation of the hydrometeorology across complex terrain, in a way that has never before been possible. Research that utilizes this technology to further investigate multiple storms, spanning a variety of snow climates, would enhance our understanding of precipitation mechanisms. Ultimately, this would improve mountain precipitation representation within statistical methods, weather models, and climate models, directly helping to improve the accuracy and precision with which we can forecast hydrometeorological extremes.
Comparison between the ASO derived accumulated snow and 10 different precipitation products including two gauge-interpolation products and eight different WRF simulations using six different microphysics and three different PBL schemes. (a-j) Spatial maps of the difference between each of the precipitation estimates and ASO; all WRF products used the RAINNC output which is akin to total precipitation. (k) total water equivalent over the Tuolumne as measured by ASO and all ten precipitation products. All WRF simulations have a “snow” and “precipitation” bar because WRF can parse liquid and frozen precipitation. The difference between the WRF precipitation and snow estimates is rain.
Brandt, W. T., Bormann, K. J., Cannon, F., Deems, J. S., Painter, T. H., Steinhoff, D. F., & Dozier, J. (2020) Quantifying the spatial variability of a snowstorm using differential airborne lidar. Water Resources Research, 56, e2019WR025331. https://doi.org/10.1029/2019WR025331
CW3E Welcomes Ellen Knappe
April 13, 2020
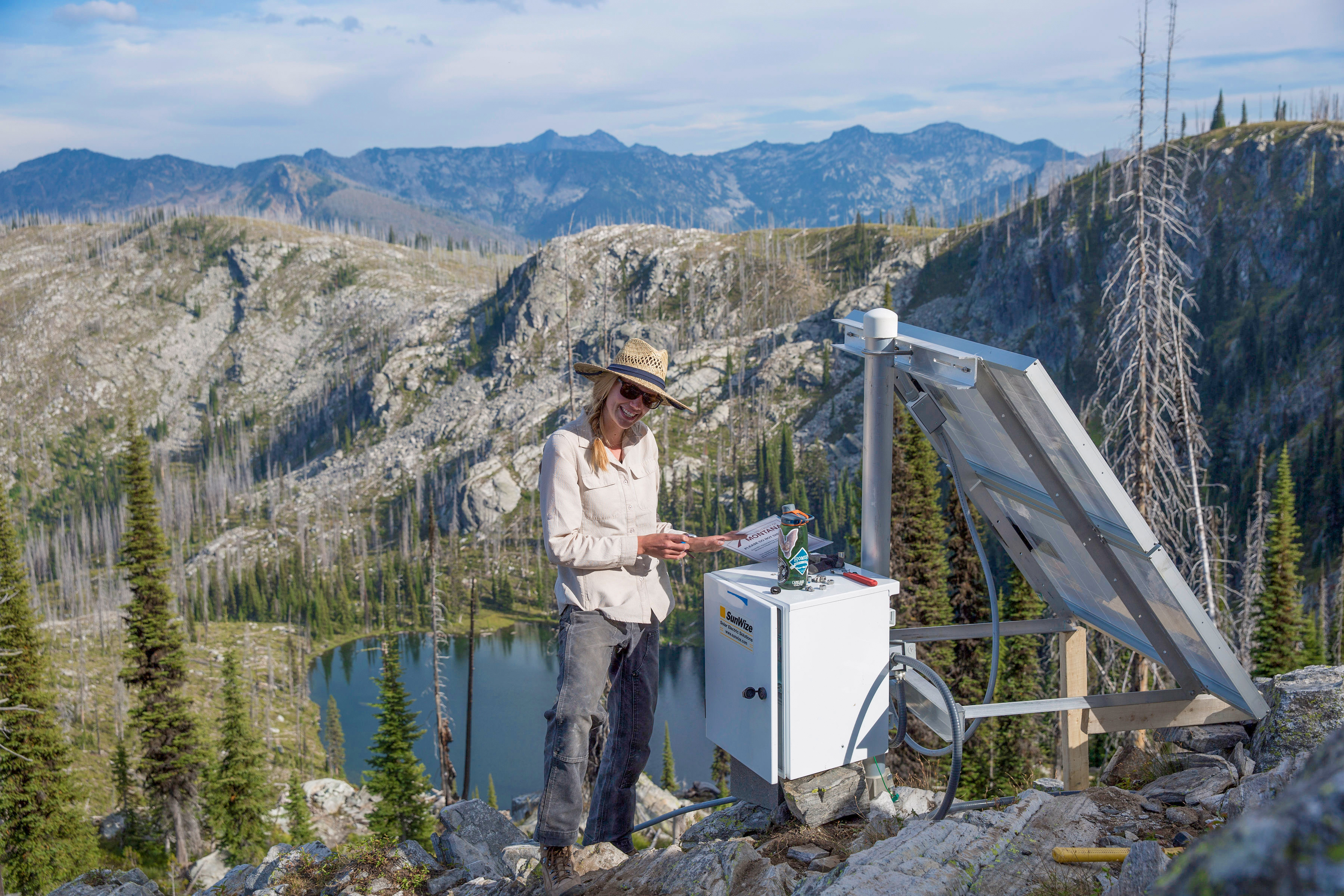
Ellen Knappe joined CW3E in February 2020 as a joint postdoc with IGPP. El finished her PhD in 2019 at the University of Montana working on constraining earth deformation using geodetic time series with Dr. Rebecca Bendick. Her research included quantifying seasonal deformation due to hydrologic loading in the mountainous watersheds in the northern Rockies. Prior to coming to Scripps Institution of Oceanography (SIO), she received her BA in Geophysics from UC Berkeley (2014) and completed a short postdoc at UM with Dr. Bendick and Dr. Payton Gardner (2020).
El’s research at UM included using geodetic observations as an independent measure of hydrologic storage in mountainous watersheds. With dense networks of stations across the US and daily observations, geodetic time series can constrain water mass at high temporal and spatial resolution. At SIO, working under advisor Adrian Borsa, she will apply and expand these methods to other regions, including the Pacific Northwest and California, working towards quantifying water storage in mountainous watersheds, water flux due to large precipitation events and tracking water through watersheds with time. El looks forward to working with CW3E to integrate geodetic observations into existing hydrologic and watershed models.
CW3E Welcomes Wen-Shu Lin
April 9, 2020

Wen-Shu Lin joined CW3E as a graduate student in the fall of 2019 after receiving her B.S. from National Taiwan University with a major in Atmospheric Science. During her undergraduate research she investigated the connections between large-scale sea surface temperatures, precipitation, and atmospheric circulations under varying stages of global warming. Her results illustrated that while there are some known patterns of future warming, regional variability among climate models is large, and at times, provides outputs of varying sign and direction. These discrepancies among climate models has sparked her interest in regional climate and she will look to continue that work at SIO and CW3E.
At CW3E, under the direction of co-advisors Joel Norris and F. Martin Ralph, she will continue her research into climate variabilities, coupled atmosphere-ocean interactions, and atmospheric dynamics. Her research will focus on closing the gap between weather and climate, specifically focused on the predictability of atmospheric rivers and regional climate on subseasonal-to-seasonal time scales.
Distribution of Landfalling Atmospheric Rivers over the U.S. West Coast during Water Year 2020: October through March Update
April 8, 2020
For a pdf of this information click here.
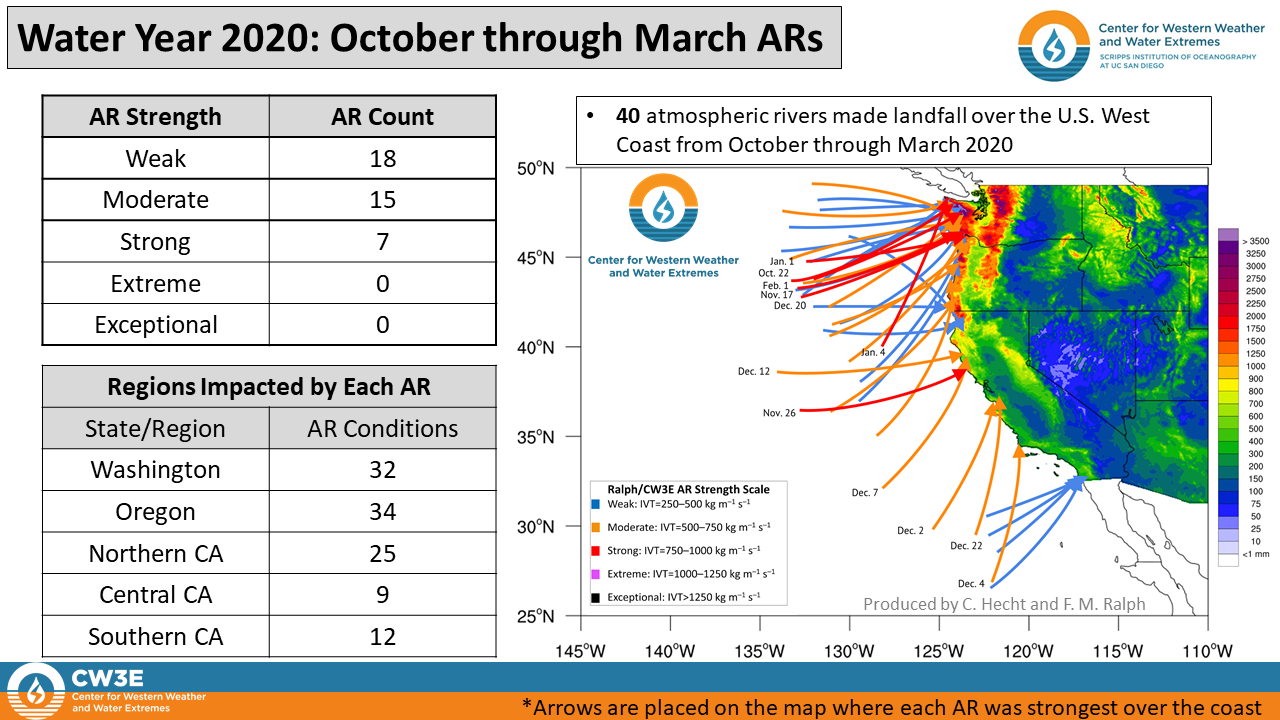
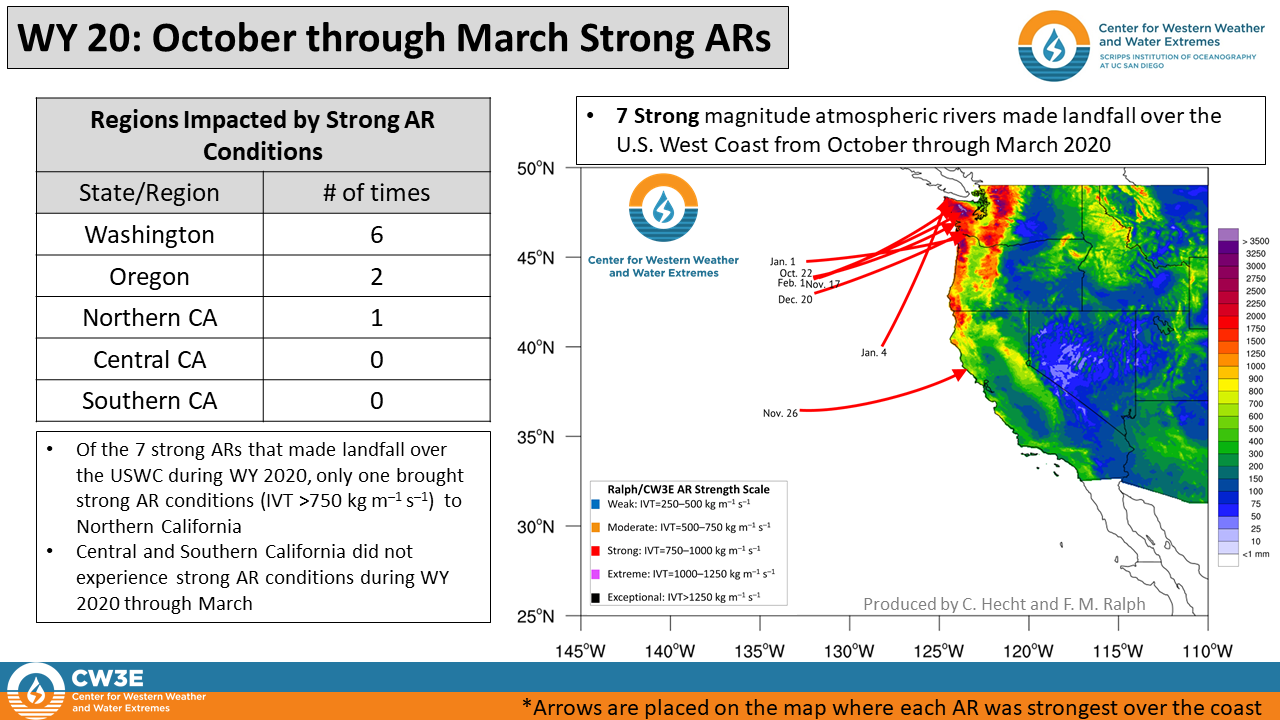
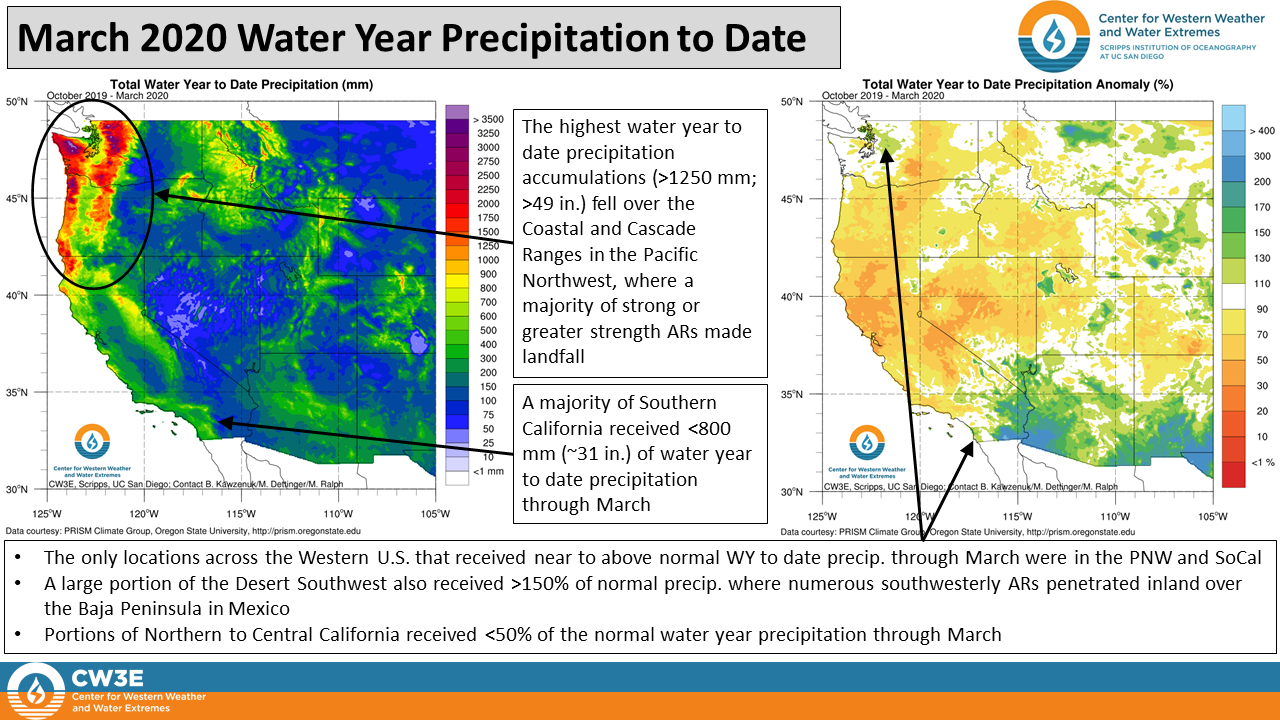
More information on water year precipitation and odd’s of reaching normal accumulations can be found here
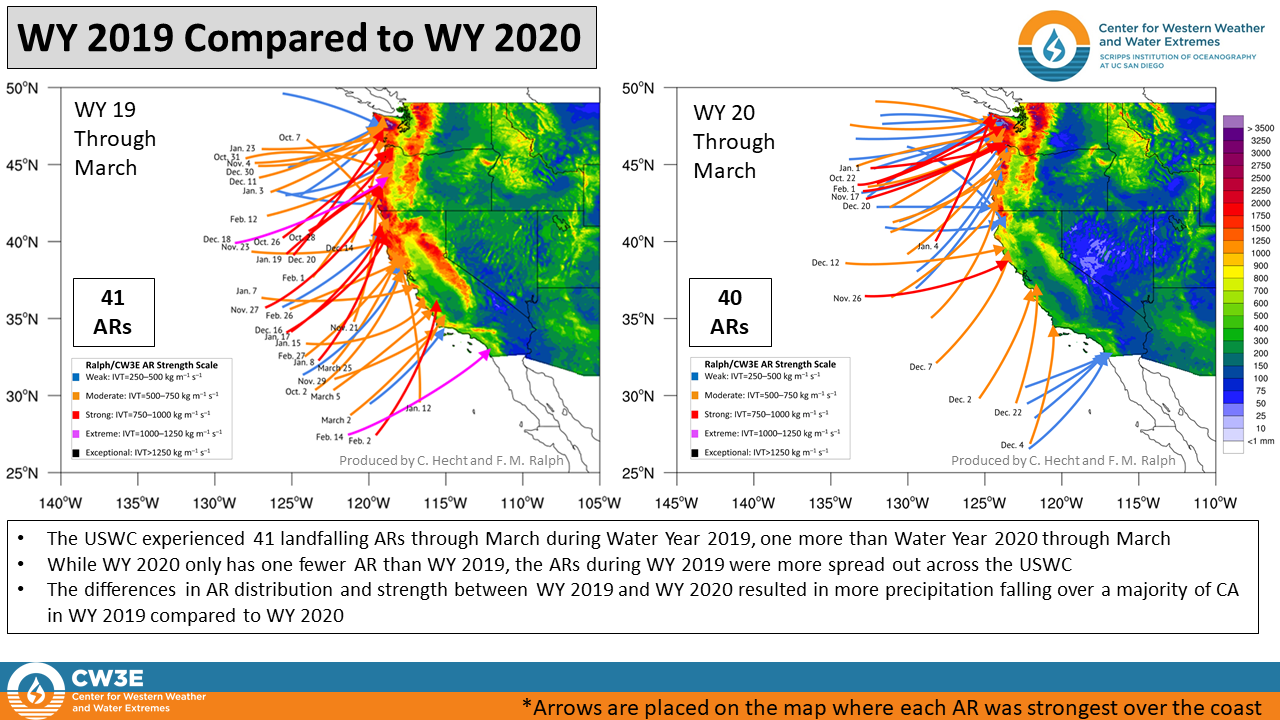
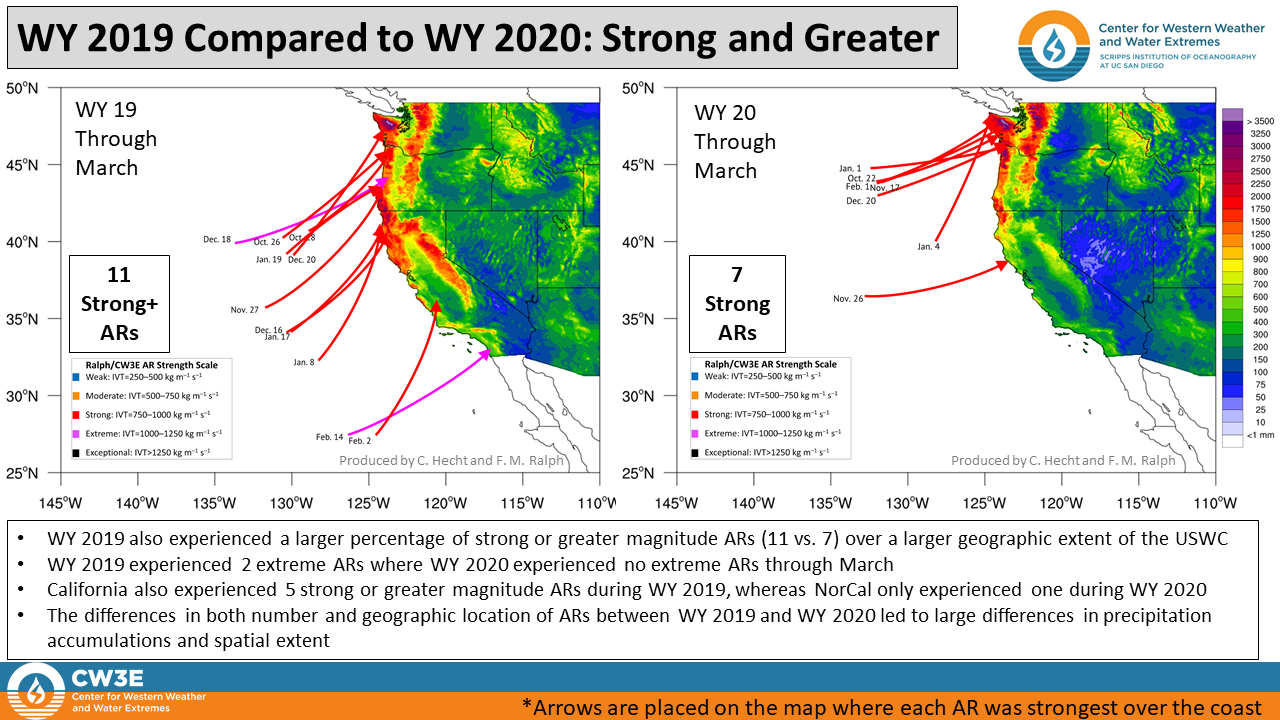
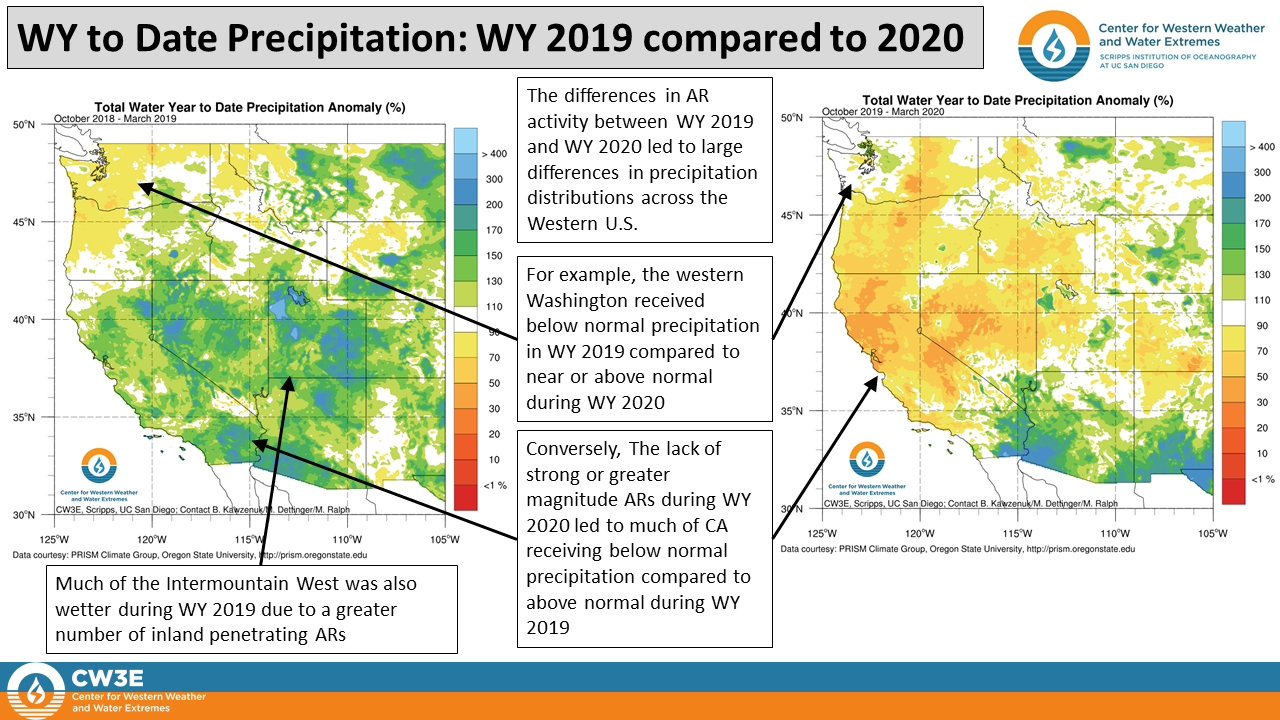
Analysis by Chad Hecht & F. Martin Ralph. This analysis is considered experimental. For questions regarding the data or methodology please contact Chad Hecht